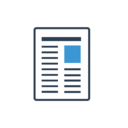
Partially non-homogeneous dynamic Bayesian networks based on Bayesian regression models with partitioned design matrices


Non-homogeneous dynamic Bayesian networks (NH-DBNs) are a popular modelling tool for learning cellular networks from time series data. In systems biology, time series are often measured under different experimental conditions, and not rarely only some network interaction parameters depend on the condition while the other parameters stay constant across conditions. For this situation, we propose a new partially NH-DBN, based on Bayesian hierarchical regression models with partitioned design matrices. With regard to our main application to semi-quantitative (immunoblot) timecourse data from mammalian target of rapamycin complex 1 (mTORC1) signalling, we also propose a Gaussian process-based method to solve the problem of non-equidistant time series measurements.
SEEK ID: https://fairdomhub.org/publications/639
DOI: 10.1093/bioinformatics/bty917
Projects: MESI-STRAT
Publication type: Journal
Journal: Bioinformatics
Editors: Jonathan Wren
Citation: Bioinformatics 35(12):2108-2117
Date Published: 1st Jun 2019
Registered Mode: by DOI

Views: 1114
Created: 11th Jan 2022 at 11:51
Last updated: 8th Dec 2022 at 17:26

This item has not yet been tagged.

None