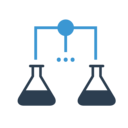
PhysiBoSS-COVID: the Boolean modelling of COVID-19 signalling pathways in multicellular simulations allows for the uncovering of mechanistic insights

Multiscale and multicellular simulation of SARS-CoV-2 infection uncover points of intervention to evade apoptosis.
Summary:
Our framework enables the simulation of the dynamics of signaling pathways that include the relevant players in SARS-CoV-2 infection, at the level of the individual cell and of the cell population. These different players encompass the virus, epithelial and immune cells. The model focuses on apoptosis and suggests two knock out alterations that force apoptosis of the infected cells.
Names of contributors and their affiliations:
Unordered draft list of authors:
Vincent Noël1, Arnau Montagud2, Miguel Ponce de Leon2, Jose Carbonell2, Thalia Diniaco2, Anna Niarakis3, Laurence Calzone1, Emmanuel Barillot1 and Alfonso Valencia2,4
1 Institut Curie, INSERM U900, Mines ParisTech, University PSL, Paris, France
2 Barcelona Supercomputing Center, Barcelona, Spain
3 Univ. Evry, University of Paris-Saclay, Evry, France
4 Institució Catalana de Recerca i Estudis Avançats (ICREA), Barcelona, Spain
Scope:
We scale-up the study of COVID-19 interaction with human epithelial cells to the populations’ level using multiscale modelling. The goal is to study how infection affects the individual cells’ phenotype, how the immune cells interfere in the process and what points of intervention can be identified using populations’ dynamics.
Multiscale modelling frameworks prove useful in integrating mechanisms that have very different time and space scales, as it is the case in the study of viral infection, human host cell demise and immune cell response. We hereby present the use of a framework, PhysiBoSS (Letort et al., 2019), to study apoptosis-evading genetic perturbation in COVID. PhysiBoSS integrates an agent-based tool for the modelling of the population of cells (PhysiCell (Ghaffarizadeh et al., 2018)) and a time-continuous Markovian simulator for intracellular models (MaBoSS (Stoll et al., 2017)) applied to the SARS-CoV-2 infection.
We leverage the efforts invested in the COVID19 Disease Maps (Ostaszewski et al., 2020) by converting a descriptive molecular map to a Boolean model and incorporating it into a populations’ model. We selected this Boolean model as a proof of concept with the aim of extending this study to the rest of the maps of the COVID-19 Disease Maps effort. Furthermore, our framework has the ability to be cell- and patient-specific: it can integrate different patients’ mutational landscape, different immune cells’ interactions and different mechanisms widely studied in the COVID19 Disease Maps.
Methodology:
Our work builds upon the PC4COVID initiative (Getz et al., 2020) that studies the dissemination of the virus and the response of immune cells using PhysiCell agent-based modelling. First, we integrated PhysiBoSS into PC4COVID to simulate pathway signaling using this multiscale modelling.
Then, we incorporated pathway-specific Boolean models to detail the interactions of virus and human cells previously generated by CaSQ (Aghamiri et al., 2020) from the COVID-19 Disease Map initiative (Ostaszewski et al., 2020). The model on which we concentrated first is a model of apoptosis in epithelial cells that includes interactions with the virus and T-cells and integrates and formalizes mechanistic knowledge, using current systems biology standards. This Boolean model is simulated using MaBoSS allowing for the study of the cells’ mechanisms and their perturbations upon mutation or virus infection, and ultimately, enabling the discovery of biomarkers and therapeutic targets with the help of our pipeline of tools (Montagud et al., 2017).
All the code, models and jupyter notebooks are available at https://github.com/vincent-noel/COVID19/ and at the project's GitLab: https://gitlab.lcsb.uni.lu/computational-modelling-and-simulation/pb4covid19.
Results:
CasQ was used to convert an apoptosis map into a model that was gathered from COVID-19 Disease Map project (https://fairdomhub.org/models/712). We modified this model to capture mechanisms such as BAX activating the apoptosome complex, and included output nodes as readouts. We also connected inputs and outputs to different variables in the population modelling, such as the Virus_inside node which depends on the number of virions inside a cell or Tcell_attached node which depends on the attachment of a T-cell to the epithelial cell.
PhysiBoSS allows to simulate the effect of genetic and environmental perturbations in multiscale models. In the present case, we used our pipeline of tools (Montagud et al., 2017) to thoroughly study all the knock out and overexpression perturbations of the Boolean model and identified two mutations that evade apoptosis in infected human host cells. The first one is the inhibition of FADD, which is a downstream actuator of FASLG reception upon T-cell activation promoting apoptosis. In the FADD knock out simulation, the cells no longer reached the CD8-T-cell-mediated apoptosis, but were still able to undergo virus-mediated apoptosis, through the activation of the apoptosome by the virus.
The second interesting mutant that evaded apoptosis is the inhibition of the virus’ M protein that inhibits AKT1 protein, promoting apoptosis. M knock out greatly limited the virus-mediated apoptosis, reducing the chemokines released in the environment, delaying the activation of the macrophages and T-cells (representative of the immune system in this model) and deferring the T-cell-mediated apoptosis.
Using this latter mutant, we further studied the dynamics of a heterogeneous cell population, which consists on a combination of WT and mutant cells in a single population. We thus combined WT and M knock out cells with different proportions (50% and 95% M knock out cells) and saw that virus-mediated apoptosis was highly reduced and that more inhibition increased the delay to the activation of the immune system.
Conclusions:
The use of PhysiBoSS (Letort et al., 2019) on COVID , provides a framework that enables testing of combined genetic and environmental perturbations, offering mechanistic insights of SARS-CoV-2 infection and dissemination among human host cells.
To help model calibration and parameter exploration, an extreme-scale model exploration platform (EMEWS (Ozik et al., 2018)) can be used to calibrate parameters for which there is scarce information and to explore high-dimensional parameter spaces to identify new hypotheses that lead to the better understanding of the SARS-CoV-2 infection and potential intervention points.
As future steps, we plan to integrate models of immune cells’ differentiation, expand the epithelial cell model, use cell-type-specific models and personalize Boolean models with patients’ data.
Finally, we are working on scaling up this work to HPC clusters to simulate complex, 3-dimensional setups with millions of cells and cell-specific models. This is due to the fact that PhysiBoSS on COVID is one of the use cases of the European HPC/Exascale Centre of Excellence in Personalized Medicine (PerMedCoE, http://permedcoe.eu/), whose purpose is to adapt multiscale modelling to supercomputing environments and to provide an easy-to-use interface to systems biology end users.
References:
Aghamiri, S. S., Singh, V., Naldi, A., Helikar, T., Soliman, S., & Niarakis, A. (2020). Automated inference of Boolean models from molecular interaction maps using CaSQ. Bioinformatics (Oxford, England). https://doi.org/10.1093/bioinformatics/btaa484
Getz, M., Wang, Y., An, G., Becker, A., Cockrell, C., Collier, N., Craig, M., Davis, C. L., Faeder, J., Versypt, A. N. F., Gianlupi, J. F., Glazier, J. A., Hamis, S., Heiland, R., Hillen, T., Hou, D., Islam, M. A., Jenner, A., Kurtoglu, F., … Macklin, P. (2020). Rapid community-driven development of a SARS-CoV-2 tissue simulator. BioRxiv, 2020.04.02.019075. https://doi.org/10.1101/2020.04.02.019075
Ghaffarizadeh, A., Heiland, R., Friedman, S. H., Mumenthaler, S. M., & Macklin, P. (2018). PhysiCell: An open source physics-based cell simulator for 3-D multicellular systems. PLOS Computational Biology, 14(2), e1005991. https://doi.org/10.1371/journal.pcbi.1005991
Letort, G., Montagud, A., Stoll, G., Heiland, R., Barillot, E., Macklin, P., Zinovyev, A., & Calzone, L. (2019). PhysiBoSS: A multi-scale agent-based modelling framework integrating physical dimension and cell signalling. Bioinformatics, bty766. https://doi.org/10.1093/bioinformatics/bty766
Montagud, A., Traynard, P., Martignetti, L., Bonnet, E., Barillot, E., Zinovyev, A., & Calzone, L. (2017). Conceptual and computational framework for logical modelling of biological networks deregulated in diseases. Briefings in Bioinformatics, bbx163. https://doi.org/10.1093/bib/bbx163
Ostaszewski, M., Niarakis, A., Mazein, A., Kuperstein, I., Phair, R., Orta-Resendiz, A., Singh, V., Aghamiri, S. S., Acencio, M. L., Glaab, E., Ruepp, A., Fobo, G., Montrone, C., Brauner, B., Frishman, G., Gomez, L. C. M., Somers, J., Hoch, M., Gupta, S. K., … Community, the C.-19 D. M. (2020). COVID-19 Disease Map, a computational knowledge repository of SARS-CoV-2 virus-host interaction mechanisms. BioRxiv, 2020.10.26.356014. https://doi.org/10.1101/2020.10.26.356014
Ozik, J., Collier, N., Wozniak, J. M., Macal, C., Cockrell, C., Friedman, S. H., Ghaffarizadeh, A., Heiland, R., An, G., & Macklin, P. (2018). High-throughput cancer hypothesis testing with an integrated PhysiCell-EMEWS workflow. BMC Bioinformatics, 19(18), 483. https://doi.org/10.1186/s12859-018-2510-x
Stoll, G., Caron, B., Viara, E., Dugourd, A., Zinovyev, A., Naldi, A., Kroemer, G., Barillot, E., & Calzone, L. (2017). MaBoSS 2.0: An environment for stochastic Boolean modeling. Bioinformatics, 33(14), 2226–2228. https://doi.org/10.1093/bioinformatics/btx123
SEEK ID: https://fairdomhub.org/investigations/447
Projects: COVID-19 Disease Map
Investigation position:

Creators
Additional credit
Jose Carbonell, Thalia Diniaco, Emanuel Barillot
Submitter
Views: 1727
Created: 10th Feb 2021 at 10:45
Last updated: 13th Aug 2021 at 10:12

This item has not yet been tagged.